Turfgrass Seed - Could it be more helpful?
Turfgrass Seed provides, for different turfgrass species maintained under varying mowing regimes and with simulated wear, tables of performance for individual cultivars. Performance is measured in terms of various parameters that include shoot density, fineness of leaf, resistance to red thread etc. For perennial ryegrass there are, in the 2016 edition, 114 cultivars evaluated for performance at a 'lawns' mowing height of 10 to 15mm and 105 cultivars at a 'sports' mowing height of 25mm. Most of these (93) have been evaluated at both mowing heights and so the names of some cultivars appear in more than one table. The equivalent data for a range of other species is also provided and it is these tables that comprise the majority of the booklet.
I first became familiar with the overall approach to the production of Turfgrass Seed as long ago as 1989 when I was working alongside the people who produced it. Since then, while a major overhaul took place around 2002 when the BSPB took over the running of it from STRI, the basic measurements and evaluation techniques appear to have altered only slightly. The booklet provides a huge amount of information but, being paid for by the seed companies themselves, it might be said that it serves their needs rather more effectively than those of the end users of grass seed.
For example, within the industry, a great deal of attention is paid to the ranking of individual cultivars, how high or low in the tables a cultivar might be. This informs the corporate competition that exists among seed producers, but is of questionable merit for grounds-people and greenkeepers. Identifying from the booklet which cultivar an individual might choose to serve a particular purpose is by no means straightforward and seems to require a good deal of understanding of both turfgrass agronomy and, indeed, of statistics.
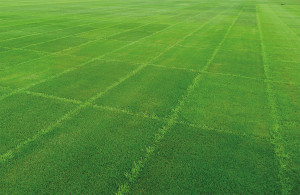
Considering the data has been collected year upon year, and that it has been re-processed and reprinted each and every January, the amount of accumulated data on turfgrass cultivar development and performance must be truly enormous. With such consistent forms of data, collected over so long a timescale, it will be possible to examine individual cultivar performance under differing annual weather variations or even perhaps to derive more general information pertaining, for example, to climate change. What I am concerned with here, however, is how the booklet could be made to provide practical information on cultivar performance that is more easily drawn out by the lay person, and I would like to describe a way in which this can be done.
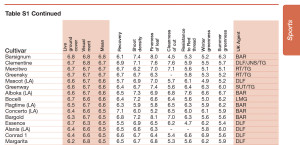
If you plot the values of, for example, 'visual merit' against those of, say, 'fineness of leaf' you get what is called a scattergram. That particular scattergram, for all of the sports ryegrass cultivars, is shown in Figure 2.
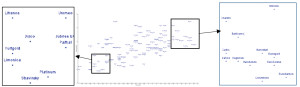
Now we could plot similar scattergrams for all of the possible pairs of measured criteria. 'Live ground cover' against 'shoot density', for example, or 'cleanness of cut' against 'resistance to red thread' and all sorts of interesting comparisons could be made. Because most of the measurements are in some way related to one another (greater visual merit is generally associated with higher shoot density, for example), it is possible, using a clever statistical technique, to present most of the variability in the data in just one scattergram. To achieve a more comprehensive analysis of cultivar performance, I combined the data for sports maintenance with those for lawns (using only those cultivars that were represented in both tables) and the resultant scattergram is shown in Figure 3. This accounts for 67% of all of the variation present in tables S1 and L1.
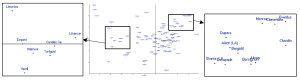
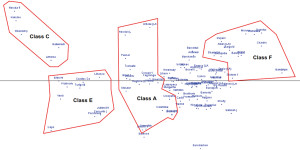
Using another clever statistical technique, and again one that takes into account all of the data available, it is possible to place the cultivars into groups of generally similar characteristics. This is not dissimilar to assigning stars to particular constellations or the streaming of school pupils into classes on the basis of their performance in different subjects, French, maths etc. You can dictate at the start how many classes you would like the data to be divided up into. For my example of the combined data from cultivars represented in both tables S1 and L1, I have chosen to identify 6 classes.
Each of those 6 classes represents a cluster of cultivars that are similar to one another and therefore occupy a particular space in the scatter distribution of cultivars as shown in Figure 3. The space occupied by 4 of these classes are shown in Figure 4.
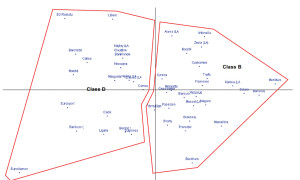
On the axis 1-2 scattergram the separation of Classes B and D is not so clear as the others. Another axis, axis 3, accounted for a further 11 % of the variation in the data. This may be thought of as extending vertically through the page so we are, in effect, looking at our cultivar constellation from the side. In that way, the separation of the cultivars not shown as belonging to a particular class in Figure 4 becomes much more distinct and the separation of classes B and D along this third axis is shown in Figure 5.
Now we have divided the perennial ryegrass cultivars into 6 fairly distinct classes and the cultivars in each class should all share similar characteristics. We can now look at what those characteristics actually are and we can assign named cultivars to each class. The constituent cultivars in each of the 6 classes are indicated in Table 1.
Another problem with the presentation of the data in the booklet is that the actual performance scores, 7.1, 6.3, 8.5 etc., and which have all been standardised, actually vary very little one with another. The measures for 'visual merit' in sports, for example, vary only between 5.3 and 8.1, a range of just 2.8. This gives little indication of how significant any variations that are presented actually are.
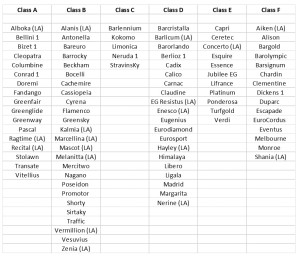
So, each of our 6 classes has an average score for each of the measured variables and those scores, expressed as a percentage of the range actually occurring, are shown in Table 2.
This gives rise to the possibility of describing the performance of the classes of cultivars, not so much in numbers but in words that users may easily understand. Such descriptions have, I think, the potential to be much more widely understood and utilised. From the data in Table 2, they might read something like this:
-
Class A: Relatively low shoot density, fineness of leaf and visual merit and very poor winter and summer greenness. Good red thread resistance, especially at lawn height.
- Class B: Good shoot density, leaf fineness and visual merit. Resistant to red thread at close mowing, but not at sports height. Cuts cleanly, but very poor winter and summer greenness.
- Class C: Excellent winter and summer greenness and red thread resistance. Very poor shoot density, leaf fineness and visual merit, cutting only poorly.
- Class D: Very good density, leaf fineness and visual merit. Very poor red thread resistance and very poor winter and summer greenness and cutting.
- Class E: Very poor in all respects with low to moderate red thread resistance.
- Class F: Excellent shoot density, leaf fineness and visual merit and cut quality. Good red thread resistance at lawn height, reduced red thread resistance for sports. Very poor winter and summer greenness.
No classification can be absolutely perfect and this analysis of course showed variation, or noise, within and between groups. That variation tended to be greatest in relation to the measures of red thread resistance and cleanness of cut. In the case of Class E, summer greenness was also somewhat inconsistent. It is not unlikely that these particular measures are prone to greater error due to their more subjective nature, but lesser inconsistencies were noted among the other measures also.

Given that the amount of data that is now available is so huge, any classification along these lines and using all of the available data collected over time would be fairly definitive. Whilst it could be re-worked each year as new cultivars become available, it is unlikely that its general form would vary a great deal one year to the next. It follows, therefore, that new cultivars could be located in a particular class simply on the basis of the set of data that would come with it. For example, the cultivar Dylan was tested for the 2016 booklet for lawns, but not for sports and, therefore, was not used in the generation of this classification. Dylan returned the data in L1 shown in Table 3 above.
Although it may not be immediately apparent, these data indicate that Dylan scores highly for shoot density, fineness of leaf, visual merit and cleanness of cut, but is only poorly resistant to red thread and has low winter and summer greenness. Dylan's scores, fed into the classification, suggest that this cultivar belongs in class F of our example classification.
If data obtained for the same cultivars over several years were to be analysed in this way, then individual cultivars would be found to move around within the space described by the derived axes as shown in Figure 3, Figure 4 and Figure 5; three years of data would present each cultivar as 3 different points that could be joined by a line representing the passage of time. The subsequent classification would then indicate how and to what extent particular cultivars responded, for example, to varying seasonal conditions.

This approach to data analysis is not especially new and it has been used widely in other fields, notably archaeology and some social sciences where data from very many items is collected and needs to be analysed.
What makes it especially possible is that, since the development of these statistical techniques in the middle of the last century, advances in the power of computers allows us to undertake these sorts of analyses on very large data sets indeed. In this respect, the possibilities afforded by the many years of turfgrass evaluation that have taken place to date are especially exciting.
I look forward to analysing the Turfgrass Seed data more fully in this way and, hopefully, the resulting classification will be of practical value to many in the turfgrass industry.
The Turfgrass Seed booklet for 2017 may be downloaded from the BSPB website.
Dr Tim Lodge, Director, Agrostis Turf Consultancy Ltd.
www.agrostis.co.uk